Fast Sort
2018-12-17
In my last post I was benchmarking various sorts against each other to test a couple of radix algorithms. https://blog.computersarehard.net/2018/12/sort-benchmarks-and-modern-processors.html . Radix Sort came out the clear winner for large data sizes (as we would expect), but that experiment left some questions.
In particular, on small arrays (less than ~100 elements in size), Radix Sort performed quite poorly. I posited a couple of theories. Maybe it's prefetching and branch prediction, or maybe it's all of the passes over the radix-array itself that costs more (as a friend of mine suggested). If the former is the main factor than we should see little performance difference as the radix-size changes. If the latter is the main factor then as the radix-size goes up our runtimes on these small arrays should shoot through the roof.
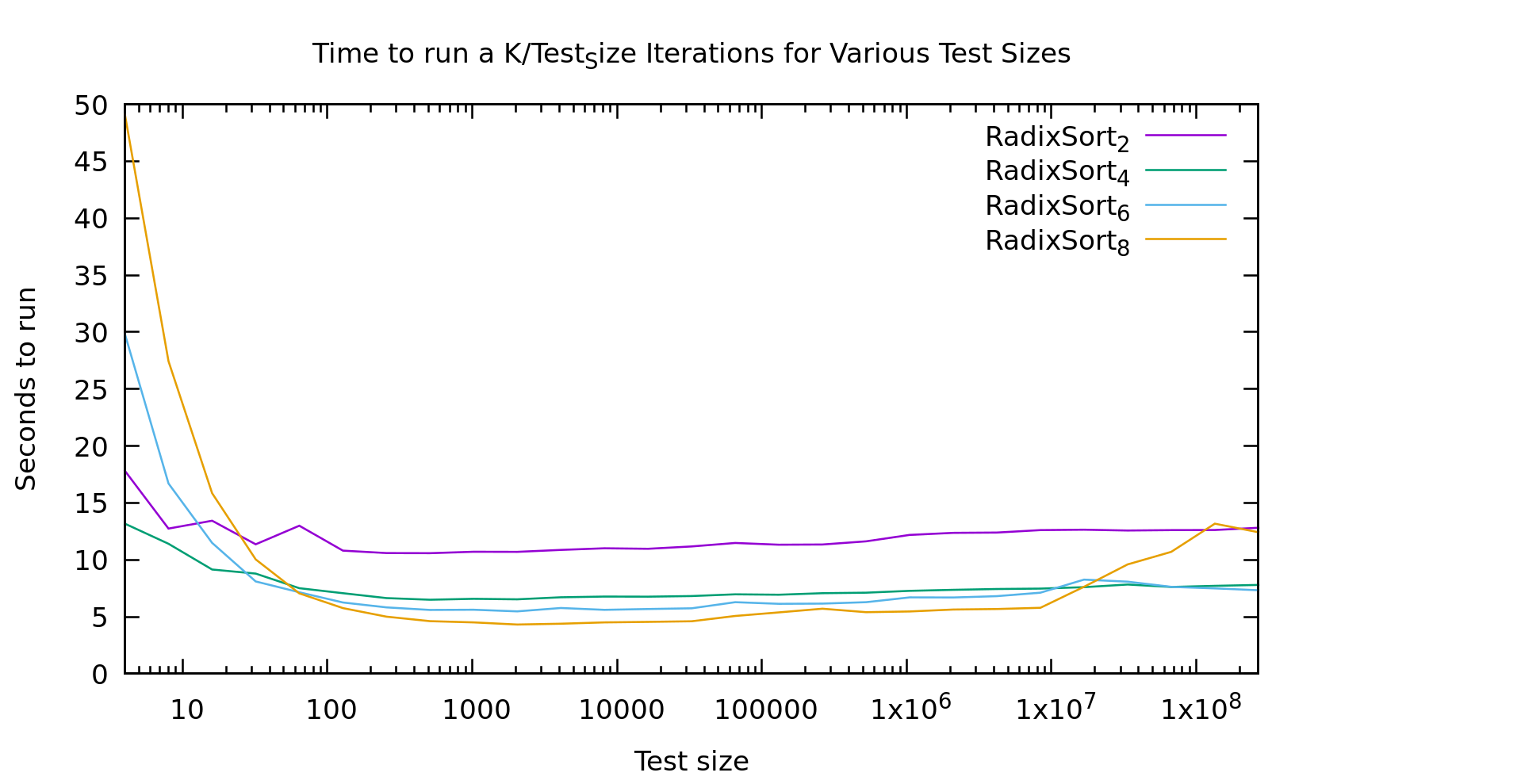
As you'll recall from my last post, every point on this graph involves sorting the same number of elements. The X axis indicates the length N of the array sorted, but it is then sorted K times where N*K = a constnant. This way this graph shows nonlinearities in the algorithms as they change with N. The subscript for each algorithm is the number of *bits* in the radix, so the actual radix-size is 2^K (e.g. 256 for the yellow line). Note that the constant is not the same as my last post, so please don't cross-compare the graphs.
This graph gives us a pretty clear answer. Our slow performance on small arrays is definitely the radix array. With a radix of 256 it doesn't start to look linear until around 1000 elements, and doesn't beat a radix of 2 until ~20 elements.
You're probably wondering about the weirdness out on the right. Testing on any larger arrays gets somewhat time-consuming quickly. Logarithmic graphs are annoying that way. That said, I generated several graphs like this one, and all had similar artifacts towards the right, especially for larger radix sizes. But it looks pretty noisy overall. My current guess is caching behavior. The machine I'm testing on is far from sanitary, lots of other stuff is going on in the background. It could be that as radix sort uses nearly the entire cache it becomes highly sensitive to other workloads. A larger radix might have more opportunity for chunks of the radix array to get kicked out repeatedly and thus be more sensitive.
Mixed sort
We saw in my last post that radix sort is extremely valuable for larger data-sizes, in fact, it beat out eeverything else by around 40 elements. On the other hand, we also saw that it has pretty bad behavior on really small arrays. Those results were found with a radix size of 32 (or 5 bits, which would land between the blue and green lines on todays graph).
From this new experiment we see that there is a significant cost to larger radix sizes. For most uses on modern computers 256 integers is a non-issue memory-wise. But, with a large radix our performance is abysmal on really small arrays. A standard solution to this type of problem is to blend algorithms (this is frequently done with quicksort, using selection sort for extremely small arrays). Heapsort and mergesort both are stable sorts as well so they seem like good candidates. Lets see how they compare.
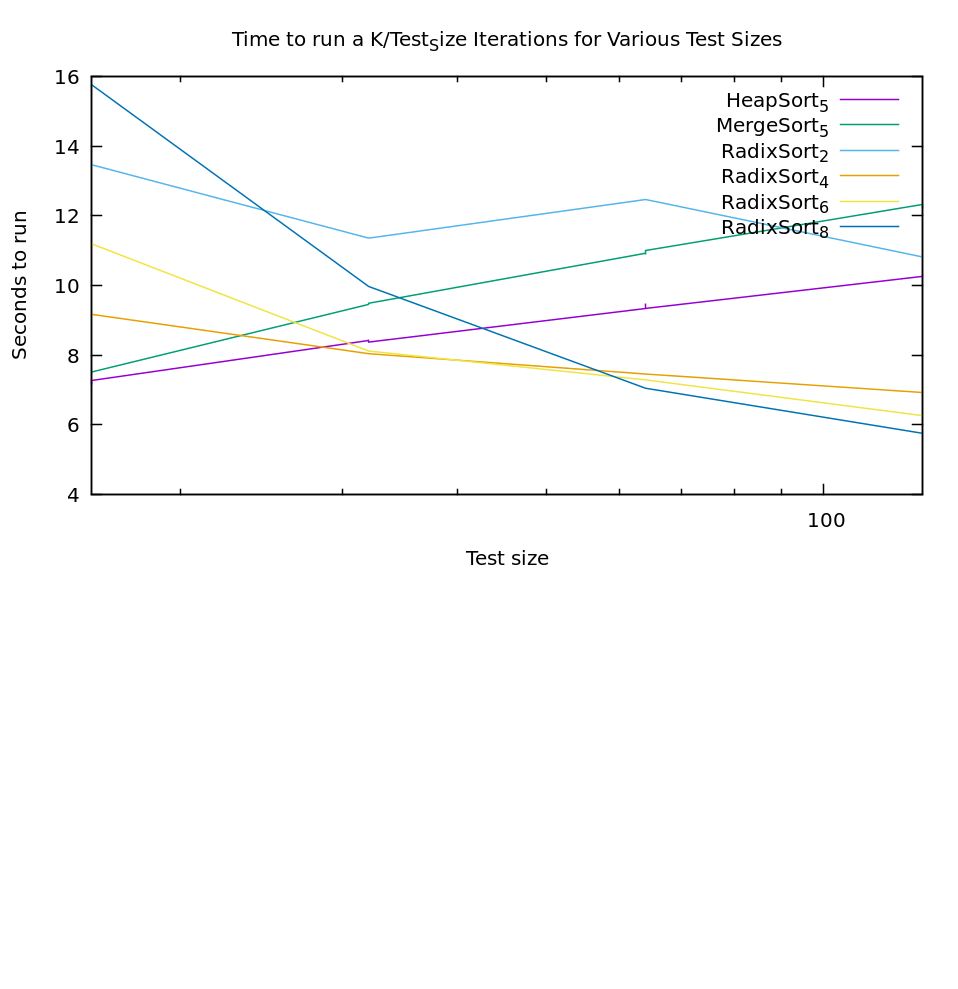
The graph is a bit ugly because I didn't do a ton of points, but the results are still pretty clear. If we just take the best algorithm at every point it looks like we should use heapsort <20, then radix sort with 4 bits up to 30, then radix sort with 6 bits up to 60, and then radix sort with 8 bits. No doubt if I tested larger radix sizes this trend would continue.
Of course, if our conditional choice gets to complex it's cost becomes a problem as well. To make radix sort fast it's compiled with the radix size as a template parameter, so each switch is going to tresh code cash, etc. If you're getting THAT picky you'll be benchmarking for your specific use-case anyway. So for general use I would propose that we keep it simple. Heapsort up to about 30, and then radix sort with 6 bits above that is a nice simple algorithm that gets us pretty close to our ideal speeds. It certainly wipes the floor with any other single algorithm. We'll call our new mixed algorithm fast sort.
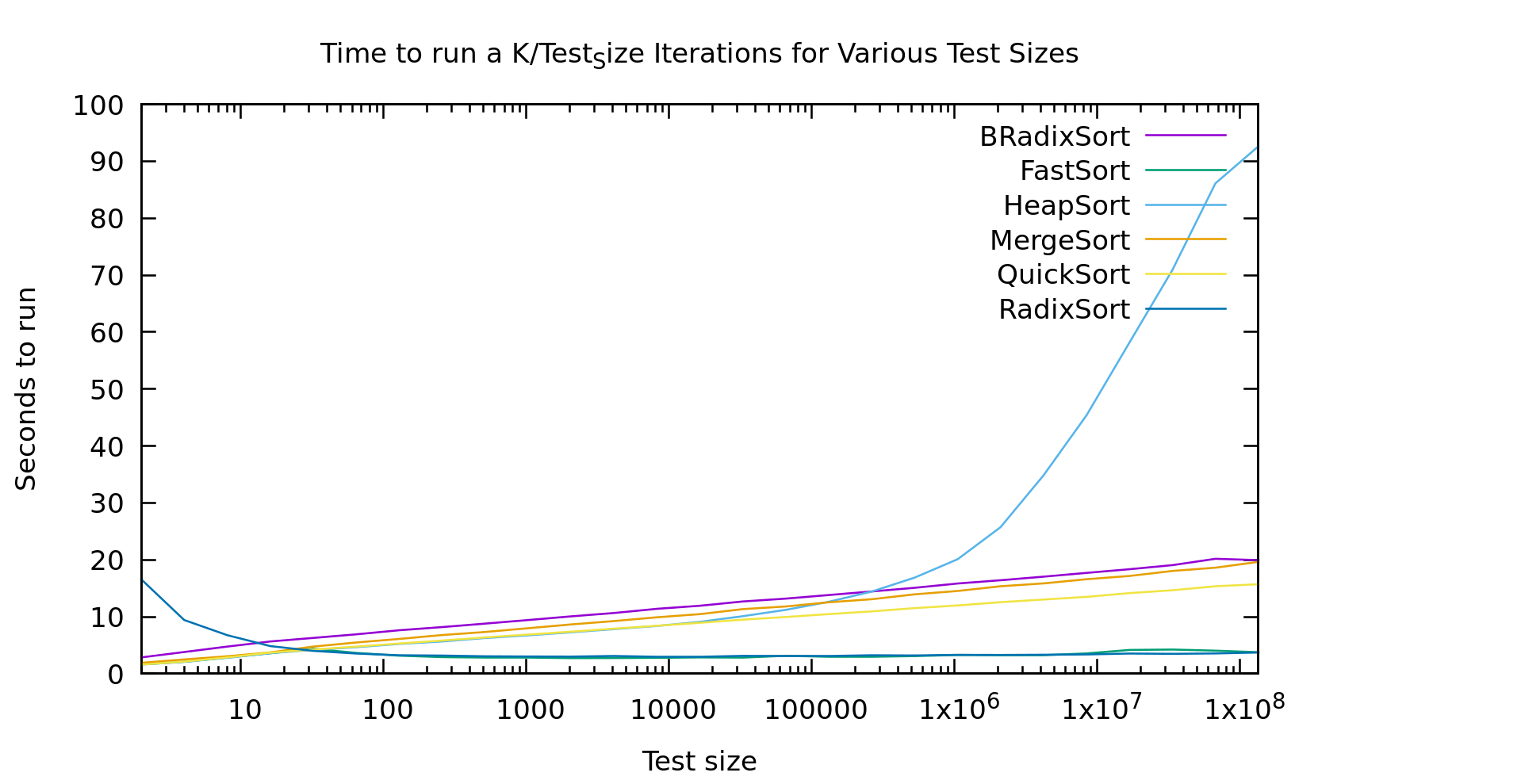
It's a little hard to see the green line that is fastsort, but that's because it performs exactly how we would hope, like heapsort up until 30, and then like radix sort with a 6 bit radix.
Conclusion
Discussions of sort speeds are usually theoretical (how many comparisons are being done). In reality, as we showed with AVL trees being just as fast or faster than Red Black trees, moves and cache behavior play at least as big of a role as comparison count. Most of the literature I've seen says heapsort is not a good choice for speed alone, while here we've found it to be a very good option for smaller arrays. Our final fast sort algorithm differs from anything you are likely to find in a textbook, yet is an extremely performant, and by happinstance stable, sorting algorithm.
There are of course caveats to these conclusions. As noted in earlier posts, these are my implementations, it's always possible (even probable) that I'm farther from the ideal implementation for one algorithm than another. Additionally our fast sort has one rather annoying property drastically limiting it's utility. Our radix algorithm has to work on the type being sorted. At the moment it's written for unsigned types, but it would have to be changed for signed types, strings, integral types larger than size_t, etc. With comparison-based sorts you can sort largest-to-smallest simply by swaping the comparitor. With radix sort (and thus fast sort) significantly less trivial adjustements have to be made to the sort itself if you don't want to have to reverse it in a seperate pass. In practice, these limitations are probably why radix sort gets so little attention despite it's obvious strengths. The details can't be abstracted away as cleanly as comparison based sorts.
Sort Benchmarks and Modern Processors
2018-12-13
A couple of days ago I realized I'd never actually sat down and written a Radix Sort. For the Computer Scientists among us, we spent a lot of time in school learning about various comparison based sorts (quick sort, selection sort, merge sort, etc.), and how they compare to the theoretical lower bound. Radix sort is mentioned as interesting in that, since it's not comparison based, it can beat those bounds. They vaguely explain it, and move on.
In practice MOST sorting in actual code is done using quick sort, due to it's average runtime. I've ranted on this blog before about average vs. worst-case, and how I think software engineers tend to greatly over-emphasis average case... but even putting that aside, take a look at this graph.
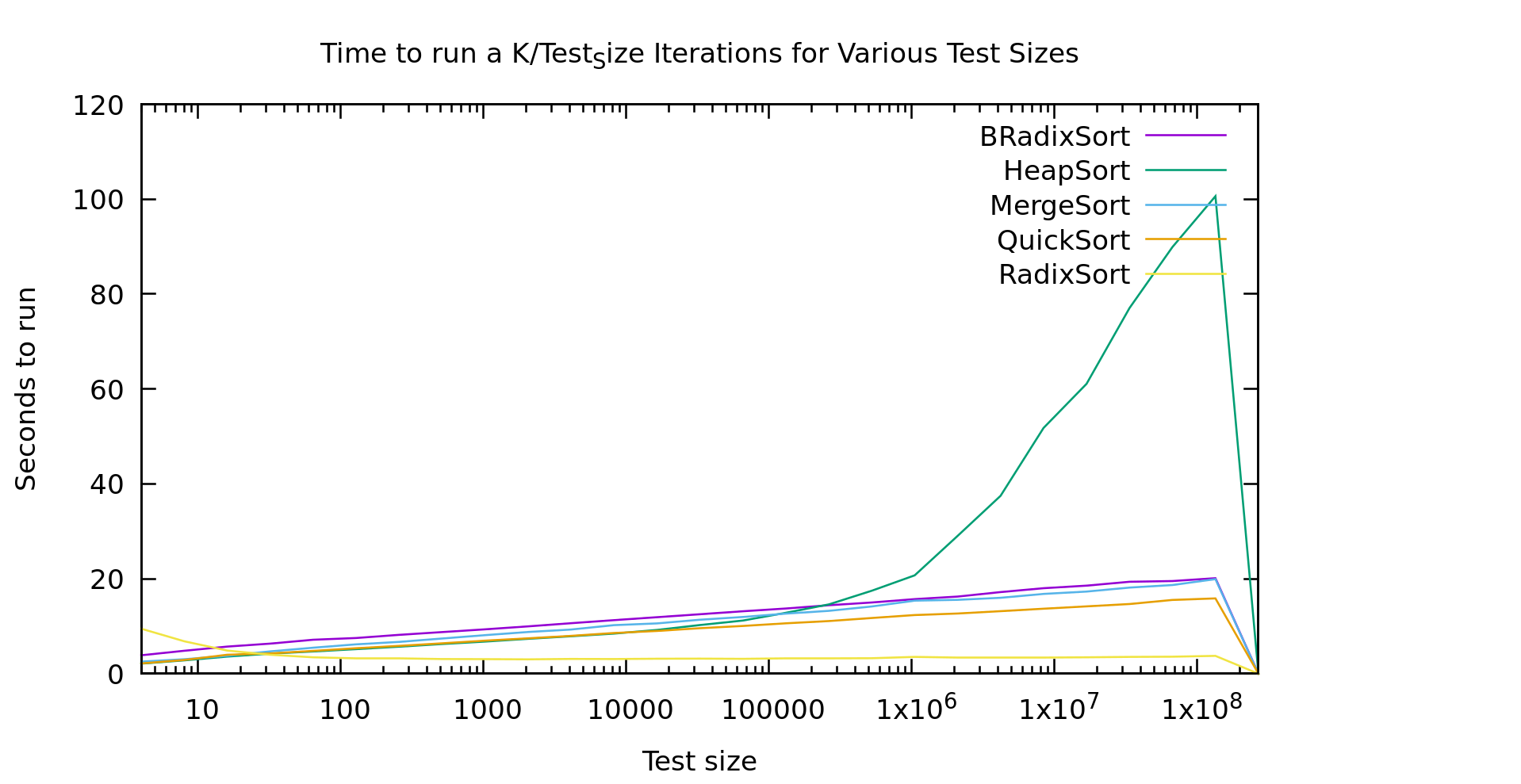
BRadixSort is a most-significant-bit first in-place binary radix sort. RadixSort is a least-significant-bit first radix sort using 32 buckets. Also, just for disclosure this is sorting unsigned 32 bit ints on my old Intel Core i7-4600U laptop.
I've shown graphs like this on this blog before, but I think I explained them poorly. Every point on this graph involves an algorithm running on the same TOTAL number of elements. The difference as you go right is that it's sorting larger and larger arrays, while doing it fewer and fewer times. This way the graph drops out the effects of just sorting more elements, and instead shows the non-linearities in varous algorithms as they work with larger and larger arrays.
Make sense?
Now. Most of these results are about what you'd expect if you've got a background in this field. Except three oddities.
- Heap Sort seems to hit a threshold list-size where it starts performing really poorly. It was looking similar to the other O(nlog(n)) algorithms, then it takes off for the skies.
- Take a look at Radix Sort below 100 elements. It gets *faster* as it runs on more elements.
- Why isn't Binary Radix Sort behaving like it's time is O(nlog(k)) like Radix Sort is?
Heap Sort
With heap Sort I'm I think this is related to cache sizes. We all know that quick sort and merge sort are preferred over heapsort due to constant factors right? Well sure, but here we see heap sort is actually faster than merge sort for smaller lists (this could actually potentially be useful, since it's also in-place and stable). I think the problem is that as heaps get bigger you completely lose adjascency between a node and it's children. This means basically every access is random, no prefetching and caching advantages. My machine has a 4MB cache. It falls apart just about the time it starts cache-thrashing, while other algorithms are taking advantage of prefetch logic by running liner through the data
Radix Sort
Radix Sort should take O(n*log(k)) where k is the largest element we're sorting. You can also think of log(k) as the number of bits in the elements we're sorting. This is of course completely linear in N, and the graph shows almost exactly that. Except that weird slope at the start.
That bump at the start has a couple possible causes. It could be branch prediction failing us horribly, or it could be walking that 32-bucket list twice. It might be interesting to try and pick it apart.
To explain why branchprediction makes sense. When we sort a tiny list over and over and over again, our algorithm isn't doing anything terribly predictable. So, very likely branch prediction *correctly* guesses that we'll loop basically every time, and never break the loop. If we then break that loop after only looking at 4 elements, we get a complete pipeline dump every 4 elements... ouch. Radix Sort scans both the data, and it's index array twice per pass. It does this for log(k)/buckets passes. But, each pass goes straight through list. This means LOTS of loops to mispredict, while on big lists prefetching logic knows *exactly* what we're doing, so it's able to pull in the data before we need it. Given no other factors slowing it down it actually gets faster on larger arrays, where prediction is basically perfect.
Binary Radix Sort
Binary radix is supposed to have the same time complexity as Radix Sort, but that graph doesn't look "linear in N' at all. If it was linear in N it would be a flat line like Radix Sort is. Here's my guess as to what's going on. It's true that Binary Radix Sort will take at MOST log_2(K) passes. But, that's a lot of passes. In fact, in practice log(K) is usually larger than log(N). After all, we usually work with numbers the same size or larger than the size of our address space right? Even if we limit K to 32 bits, it's still larger than most lists we'd sort on a single machine.
Binary Radix Sort is also capable of terminating early. Since it's recursive, at some point a sublist only has size 1 and there's no need to keep iterating through the bits at that point. This means the average runtime is actually more like N*min(log(N),log(K)). For every list in the graph shown here Log(N) < Log(K) so it's effectively an Nlog(N) algorithm. My guess is that if I did another 4 or so points we'd see that line go flat, but I'm just not sorting big enough arrays ('cause I don't want to wait that long).
Conclusion
There are several takeaways here:
- People should really be using RadixSort more (but based on my results, probably not for short lists, call out to selection or quicksort if it's short, smaller radicies might do better). Comparison based sorts are useful when your sorting needs to be super-generic, but in reality we are almost always sorting numbers or strings.
- HeapSort is underappreciated for small data sets. It doesn't diverge from quicksort until around 10k elements; Yet, unlike quicksort, heapsort is guaranteed O(nlog(n)). Plus it's stable as a bonus, and in place unlike mergesort.
- BinaryRadix has a place as well. While my version isn't stable, it is in place, and it's theoretical worst-case is O(Nlog(N)). The only other commonly used algorithm with those 2 properties is heapsort. While it's a bit slower than heapsort on small lists, it destroys it as heapsort runs afoul of the bad cache behavior. As we discussed it should even go flat at some point (or earlier if you have smaller keys).
One last note. I don't want to oversell my results. Please keep in mind that these are MY implementations. I may well be missing a tweak that would get a significant speedup somewhere. I spent a little time on RadixSort to get this performance. At first my constant factors were so bad it wouldn't have beaten anything until ~100k elements or so. Declaring constants as constants, replacing division ops with bit ops, etc. sped it up by around 5X. Micro-optomization is usually a waste of time, but here is where it can really pay off.
As always you can find this code on github https://github.com/multilinear/datastructures_C--11.git/ .
Blog Migration
2018-11-27
First I appolagize to anyone following me using RSS. Every post from this blog probably just appeared again in your RSS feed.
In my last post I wrote about how I was trying to reduce my usage of Google and in particular I mentioned that I'd trouble finding a good Blogger alternative. Well, I decided enough is enough and I wrote my own blogging platform. Years ago I wrote a simple static website generation library for my website www.smalladventures.net . I didn't want to think about all of the security problems, hosting costs, etc. associated with all of the dynamic stuff available out there. They're a little hacky, but they work. So, I adapted that library for use for blogging as well. I just got it working, so I haven't pushed the changes to my already hacky htmlgen library. I hope to better compartmentalize the components before publishing.
I did give something up obviously. You'll notice a distinct lack of comment support. In reality I've hardly ever gotten any comments on any of my blogs anyway. The few comments I get could've easilly been personal email given the content. So, feel free to email me about anything you read here, see the "contact us" link at the bottom. If it's super interesting/relevent I'll probably update the post with it anyway.
Update
I've published the new htmlgen code to github https://github.com/multilinear/htmlgen.py . I've also included a simple script for processing the xml produced from downloading a blogger blog and turning it in to something htmlgen can use.
Replacing Google
2018-11-24
I recently moved in to an actual non-moving apartment (if you don't generally follow me, I lived in a pickup truck for about 3 years, traveling the country).
Anyway, this afforded me the opportunity to work on a tech project I've been meaning to tackle for some time. I've tried it before, but I wanted to take another whack at it. This project is *replacing google*. That is, finding alternatives to every Google product I use in my life.
Anyone who would read this blog already knows the reasons one might want to do this, so I won't bore you with a long rant on that, and just get on to the technical stuff. I will say quickly that security/privacy are my major concerns and given those concerns switching off Google's products isn't always a clear win. This will come up later.
Let me start with a list of Google products that I was using:
- Search (check)
- Maps (check)
- Chrome Browser (check)
- Hangouts
- Email (check)
- Webmail (check)
- Contacts (check)
- Calendar
- Drive
- Docs
- Sheets
- Voice
- Keep
- Android
- Blogger (check)
- Google Wifi
Just switch
Search
This one is easy. DuckDuckGo is an option on virtually every browser, just go to settings and switch it. The nice thing about this choice is that if you don't find what you want, you can always go to google.com anyway. At least you're only doing a few searches there instead of all of them.
Maps
For desktop use https://www.openstreetmap.org is a so-so alternative. It works pretty well for looking up distances and routes and such. What it lacks is good search functionality and as much info about local businesses. On mobile I've been using MapsWithMe for some time. It works well offline (unlike Google maps), but can't do address searches, which can be an issue.
Chrome Browser
Another easy one. I switched to Firefox. There are other options like DuckDuckGo, but firefox has the best support, usability, and security, with the least spying. This is a place where you have to consider your tradeoffs, if all of your browsing data goes straight to various companies you didn't help yourself much. I hope to write an article on browser security/privacy at some point as well.
Hangouts
Ignoring network effects (which are huge, I realize), just switch to signal and call it good. Signal supports all of the things you need (text, voice, video), and is the most secure option available. They just changed the protocol in fact to make it even harder for them to comply with any subpeana demands.
Personally, I have some issues though. Signal is available for basically every platform... but they didn't roll out a chrome OS version prior to the protocol switch, so Angie (my wife) can't use it on her laptop (Yes, it's Google as well). Additionally I have a Samsung Galaxy Tab A 7" which is stuck on Android 5 and the new Signal version wasn't backported (I can't blame them really). These are both fairly unique and weird requirements though, I recognize that... for 99.9% of people, Signal is your solution.
Hard: Self Hosted Services
Everything below is self hosted on my own machine, so I need a good connection, a domain name, port forwarding, and a good hosting machine which in my world means Linux. I'm on comcast business cable, and I got a Google wifi access point (yes... also a Google product). I set the cable router to be a bridge so the Google wifi is the endpoint/NAT, then set up port-forwarding through that. Finally I don't have a static IP. After a little research I decided on duckdns for dyn-dns. Their tooling is open and the price (free) is good. My machine is Debian, because I find it easier to run and administer than having to remove all the junk Ubuntu comes with (even on the server installs).
For the moment I'm serving off my primary laptop, since that's what I have. If my laptop is at home, I can reach it from a coffee shop on my tablet, if I have it with me, I can reach services using localhost. It's not ideal, but it works until I get another machine (I'm cheap).
As a techie, my solution is to host my own email. I did this some years ago, and gave up because you basically can't use email with google's email filtering these days. So, this time I compromised. My email goes to gmail. I use fetchmail to pop it to my laptop (using a .fetchmailrc style config), deleting the email from gmail. then I use dovecot to run an imap server, and finally thunderbird on my laptop and k9mail on my tablet. All in all it works extremely well, plus I get pgp support and all of those things too. If my machine is down, email sits in gmail until I can pop it, so if I screw up I don't miss email. Honestly, this setup was significantly easier than I expected.
Webmail
If you want webmail, this one is comparatively easy. I'm not running either right now because I don't care for webmail anyway, but I ran roundcube for a while and it worked fine. squirrelmail is an option, and if you're using NextCloud (see my next section) it has an email cient built in as well. Search won't be as good as gmail, but that's about the only flaw.
Contacts
Surprisingly after researching all the software out there there are no good standalone carddav sync servers out there. I found several options, none of which appeared stable enough and well enough designed to actually use.
So, I opted for a heavyweight solution instead. I installed NextCloud. So, to set this up the easiest way was using snapd. I installed it with apt, then used it to install NextCloud. The result is a bit odd. NextCloud gets installed in /snap, and the files aren't writable. To configure nextcloud you use the command "nextcloud.occ" located in /snap/bin (which you'll want to add to your root path). That done everything worked like a charm. A few config commands later and I had NextCloud running with ssl keys from lets-encrypt.
Since it's CardDav, basically everything supports it. I installed tbsync on thunderbird and that worked great. On my android (yup, another google product), I installed DavDroid, but you can find similar syncing apps for virutally any OS... that's the great thing about standards.
Calendar
The nice thing about the above solution, is that I get the calendar for free. Just enable it in the options, and hey, calendar! This setup *almost* interacts cleanly with Google calendar too, so you don't have to convince others to switch so you can. Pulling Calendars from google for viewing works quite well actually (and thunderbird gives you editing). Unfortunately though, Google's calDav integration is abysmal and it may be days until an update to your calendar is visible on Google. So you can't share your calendar with folks who use Google calendar... Bummer.
Syncing is CalDav based, so clients are again easy to come by. On my laptop I'm using thunderbird lighting as my client, a thunderbird calendar plugin. There is ONE little issue though. There's a bug where you *must* turn off cookies to make it work, no idea why, the developers don't know yet either (but they do know about it). On Android again DavDroid does the trick.
Drive
This is barely worth mentioning after the above. This is NextCloud's core feature. Just install the NextCloud client on each of your devices and hook it up. I'm using it for syncing my password database (But not my keyfile, which I keep distinctly on each device).
Too hard: Self Hosted services with lots of setup
Docs
Sheets
It turns out that there are a couple of products that replace these products elegantly. Callabora and OnlyOffice both seem to get good reviews and they link really cleanly with NextCloud. The problem? Hosting them is a PITA. Both of them seem to be easiest to host in a docker container, and everyone's advice is to run them on a separate machine from NextCloud if you want things to actually work. Is it possible to get these working on your little home server? Yes, I'm certain that it is, but 3 or 4 days of hacking is a LOT just for these 2 products.
Note though that if you don't require online collaboration/editing you can use some good desktop options and just share the files. abiword and gnumeric are good for most use-cases and libreoffice is there for when they aren't. For me, as i mentioned earlier, Angie uses a Chromebook, so a web-editor is a must for me to switch off of docs/sheets.
No good replacements
Google Voice + Hangouts dialer
SIP is out there and works well. I'm using diamondcard.com to get the phone number. For now I'm using it throug* Google voice while I experiment with things and get it all reliable (which means Google still know about every call I make and recieve).On my laptop (Linux) I'm using linphone. Surprisingly out of many many sip clients out there for Linux there are only a couple that aren't basically abandon-ware. Android phone's have sip built in actually, just go to the phone app and then go to the settings. You can install the phone app even on a tablet.
Okay, so then why do I have this listed under "No good replacements". The problem is having *all* the devices ring. This is possible with sip if you pay a decent amount of money, but it's not possible to do for free or almost free. Diamondcard is like $3.00 a month, which is nearly free. I can get another line for my tablet, no problem. Making them both ring though means I need a hosted PBX, and the prices for that are not in the same ballpark.
Keep
There are literally hundreds of todo list, task, and notetaking apps out there. But lets look at Keeps core features
- It handles multiple notes elegantly, letting you look at all of them. Lists are a native feature, a note is a list or not a list, making the app extremely usable without fumbling for weird format charactors.
- It's avalable on all devices, laptops included
- It's available offline on mobile devices
- It syncs between users
Android
You can buy an android device and put another OS on it, but in practice this isn't really workable. It's too much effort even for total nerds like me. You could buy an Android from someone besides Google, but then you just get worse security and no updates (like my Samsung tab A 7").
Right now I'm not aware of any good alternatives. I use my tablet for GPS (trails and roads), as a phone when on Wifi, for shopping lists in the grocery store, as a Newsreader, and a guitar tuner, as well as a laptop replacement when I left my laptop at home.
I've pre-ordered the librem 5 from purism for when it comes out, hoping that will be a reasonable alternative. But, for now a tiny laptop device is probably the best bet honestly.
Blogger
I probably haven't looked hard enough yet. Wordpress is out there, but it's security is abysmal (just look at any high-profile wordpress blog and how often it gets owned, often serving malicious content for a while unbenounced to the owner). There are a lot of heavy-weight solutions that just seem totally unnecessary. There are a lot of hosted solutions I'd have to pay for. There are a lot of lightweight solutions that appear not to work, or a so lightweight you can't tweak the look/feel at all.
I've seriously considered just writing my own software for this problem. The comment feature is rarely used anyway and then I can generate it statically using some extensions to code I've already written. In the end that may be the best direction.
Google Wifi
I mentioned earlier that I have a Google Wifi, which seems rather silly when I'm writing a blog about trying to move off Google. I actually just bought this product too. Here's why.
Wifi and router security is abysmal. Whatever device you use for NAT is the easiest device to attack from the internet, so it's the most important that it's secure. These devices are usually... well... not. Most users don't know or care if their device is secure, and have no idea when it's been rooted. As a result home routers are becoming a major source for botnets used to launch DDoS attacks, steel users information, etc. If a hacker is in your router, they can watch everything you do on the web. https ought to protect you, but it's imperfect, particularly if you're worried about so-called nation-state actors (think, any hacking group with some non-trivial resources).
Enter Google Wifi. They seem to actually care about security. They issue updates regularly which most options don't. Also the device updates itself without me having to log in to the router every couple of days and see if there's an update that needs to be installed.
I'm not going to bother listing ChromeOS because the alternatives are obvious.
Conclusion
I care about this issue a fair bit. I'm willing to go through some hassle, and even give up some functionality to make it all work. I've managed to switch off of about half of Google's products that I was using, but I find it not worth my time or money (so far) to switch off of the other half. If you have no-one else that you collaborate with regularly (friends, relatives, co-workers, or a spouse) that uses these products then you'll find the migration much easier. A lot of the lock-in occurs due to network effects which is quite frustrating. Also if you never used the collaboration features of say, docs and sheets, then switching is quite painless. Google is successful enough that their integration features vary from non-existant to poorly maintained.
So, why is this the state of the world? It's incredibly obvious, but for some reason I never realized it until I was working on this project. Good software, the best software, has to pay someone's salary. That is the only way to get enough high-quality dedicated maintainers to build solid software. Because of this the better products in the FOSS (Free and Open Source software) ecosystem are driven largely by Open Source corporations. These corporations make their money by doing hosting and support. So while the core of the software is FOSS, the part that makes it easy to run is proprietary, because that's how they make their money. End result? Lots of hobbyist products that are missing the 3 crucial features you need to make them useful (often these are things like, reliability, or actually compiling), and a few great products that are nigh-impossible to run.
In working on this I tried out a number of other options that aren't listed above, and consistently ran in poorly maintained or unmaintained software. See the discussion of replacing google Voice and SIP. Most of the clients are broken in one way or another. But, I have to admit... I'm not willing to pay, so who is?
I'll continue to work on this (like maybe getting a docs/sheets replacement online), and try and update here when I find more useful information. What I am doing, and have been doing for a while, is trying to use these products *less*. I can straight delete, or download and delete old documents and spreadsheets that don't need to be shared anymore. I can use gmail just as a caching endpoint and spam filter, and not to store my email. etc.
Rust gives me hope for the future
2018-11-16
In all of the recent political turmoil in the U.S. it's easy to get a bit down and depressed about the future. For me, a pick-me-up came from a rather surprising source... a programming language.
Now, anyone reading this post is probably enough of a computer nerd that computers are not a source of hope for the future... they are a source of the exact opposite. No computer expert can look at a programming language and not get depressed at every flaw it has. Just google "Javascript flaws" and you'll find diatribe after diatribe. C's flaws have been elevated to interview questions, I myself used to ask "what are the semantics of x++"... which would take literally 15 minutes to answer correctly. Ask a type theorist about Java's flawed generics and you'll get an hour lecture on how the designers confused top a bottom, contravariance, and why sub-typing of objects (much less generics) is a horrible idea. Alternatively, while Haskell appears to get few things *wrong* you need a PhD in category theory to understand it and like most languages that aren't fundamentally broken internally it gets relegated to the category of "useless toy".
Enter Rust
From the rust website https://www.rust-lang.org/en-US/
"Rust is a systems programming language that runs blazingly fast, prevents segfaults, and guarantees thread safety."
The end result is the first language I've ever seen that doesn't suck. As I read through the Rust book I kept being struck by how intuitive the language is. Now, I should mention that I am a little bit biased, a few of my friends, mostly with similar backgrounds, were fairly deeply involved in it's development. This means the designers have similar biases to mine.
Rust has got to be the most complex language I've ever learned... but then again, I didn't just pick up and start trying to code in C++14 without knowing C and older C++ standards first. The difference is that C++14 and similar languages don't just require learning all the keywords and what they mean, they require learning which code is defined and undefined. Ever try to actually write code that is fully defined? Sequence points are just the start of it. Just check out out the differences between char and int8_t... char (called that because it's frequently used for characters... though does completely the wrong thing with utf8 without serious effort) is assumed to alias something else, and int8_t does not. If any part of that sounded like babble... congratulations, you don't really know C++.
The reality? no-one really knows C++. It's simply too complicated a language with too many corner cases. Corollary? There is no real world software written in C++ that actually conforms to the standard. Conclusion: No real world software written in C++ is even well defined, much less *correct* by any reasonable definition besides "eh... seems to work... today... on this computer and compiler".
With rust on the other hand, while the pointer types might be a little confusing at first, the keyword definitions are all there is to learn. If your code compiles (without unsafe), the behavior is defined, and that's the end of it. No aliasing rules, no sequence points, etc. Almost all of your code can be written like this. For those rare little corners where you really need to punch through that safety, unsafe is there for you. C's semantics got screwed up by optimizing compilers, the problem was that it's definitions are a little *too* low-level (original defined by direct translation to Vax assembly instructions), so optimizing required violating the original rules and we got the crazy dance we have today. Something like SML is so divorced from the system that punching down to understand the machine-level is almost nonsense. Rust is right in between where optomizers can optomize, but the machine layout is defined enough that when you use unsafe, it just works.
It's strange to say, but as I watched the news scroll past and read the Rust book... I felt flushed with hope. Not only can software theoretically not suck, but people actually put together a tool to help us do it. A tool that itself is software that doesn't suck. Maybe, just maybe, humans can actually do this technology thing and make it all work.