Solar Inverter Monitoring
2024-07-18
I installed a hybrid solar inverter and battery bank in my home a little while ago. I have panels on the way right now, but in the meantime I've had the inverter up and running as a whole-house UPS for a little while.
But, what does this have to do with computer hackery and comp-sci theory you ask? It's a fair question and I'm getting there. The SolArk, like most modern pieces of technical hardware, comes complete with a bunch of web integration. You plug in a little WiFi dongle and your data gets whisked off to a datacenter somewhere, where you can have the privilege of accessing it in some limited ways through a mediocre web interface and maybe some annoying REST APIS. I found this unsatisfactory for a number of reasons
- This is a hybrid solar inverter. Part of it's purpose is to power my home when grid power is down, which means, network communication might also be down. When I *most* want to see my data, I'm likely to not have communication.
- I want to access the data MY way, with my own scripts
- No-one but me needs to ever see it.
- It offends my sensibilities. Why is my data traveling all over the world when I only need or want it *here*.
- I don't trust IOT devices to have properly implemented security, because, they usually don't, so it's having this thing on my network is a security hole in my network.
And my solution was this
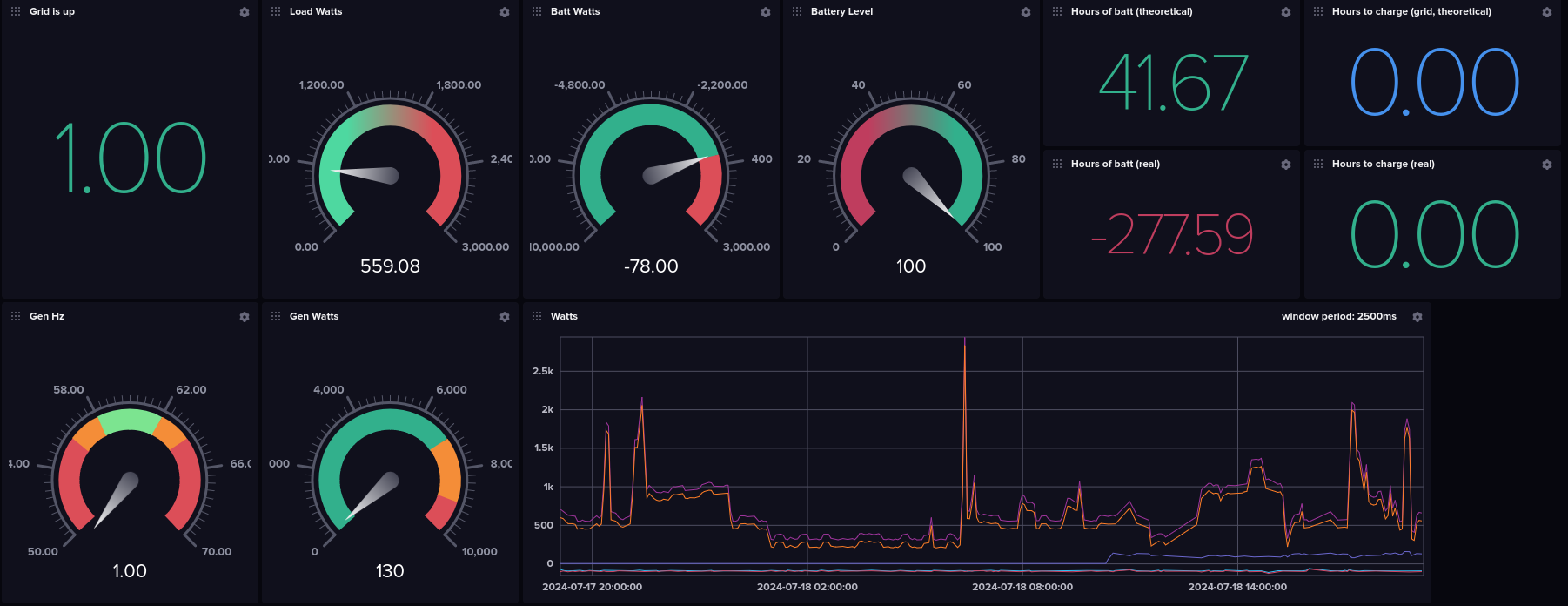
First Steps
Pulling the data
But, the Solark fit my needs really well otherwise. So, rather than whine and whinge about it I decided to see what would be required to get the data off the device myself. I thought this would be a HUGE project, but as it turns out it wasn't at all. With a little searching online I found that the solark speaks modbus, and they actually published a spec for it . You can talk to the solark over a couple of different interfaces, but I already had a USB->RS232 adapter lying around, so I plugged it in and after playing around in python for a little while I started getting data.
Logging the data
I already had an IoTaWatt for monitoring power usage in my home. It's both open-source hardware and software, and it supports pushing the resulting data into InfluxDB. So, I'm also running InfluxDB on my home server. I actually used this set of tooling to help understand my power usage so I could properly size my solar build. Anyway, the point is I already have my power usage data in InfluxDB, and it'd be great to have my solar data in the same place. So I dug up a python library for influxDB and pretty soon I had my data streaming into InfluxDB... pretty cool.
Alerting the data
Well, that's all well and good, but I have to keep looking at my dashboards. If my power goes out my WHOLE HOUSE is backed up, so there's no obvious way to even know that my power is out. That's... not great. So, I want my wife and I to get notifications somehow. I asked my wife what she'd prefer and she it should just come to our cellphones. That made sense to me. The two of us currently use the matrix chat protocol to talk to each other wherever we are. Though, we currently use an external server for this (so it won't work our communications are out), it's fully open source and I can set up and run my own server, and probably will.
So, I dug up a matrix library. This turned out to be the hardest part, especially as the first library I tried wasn't flexible enough for what I needed. I ended up moving the entire program from asynchronous, to synchronous, and then back to asynchronous again, but I eventually got it working. And, here's the result
Getting Serious
I ran that program for maybe a month and eventually got it largely working, but still felt... sketchy. In python it's hard to have any confidence in codepaths that aren't actively tested. This program is really just communication at it's heart, which means a lot of possible points of failure. But, then I hurt my foot and had a lot of time to kill, and I'd been meaning to learn rust, so I rewrote the whole thing
This was my first real project in rust and it didn't disappoint. As I expected the type safety gave me far more trust in corner cases that I'm unlikely to bother testing. The "?" operator is a really convenient cross of exceptions and error return codes. I found that it helped me think about all the error cases without adding much complexity to the code. That, combined with the single mutable reference restrictions in rust did make some functional idioms harder to use, and I definitely tried a few cute "Build up all the futures and then run them" sorts of tricks that didn't work. But, all in all it's better than any other language I've tried for this type of code.
Final notes
The final result is one of the few personal projects I could see someone else actually wanting to run, with proper configuration options and files, and all of that jazz. The real takeaway here though is that I had a problem, and I solved it, and while not *easy*, it wasn't that hard either. I run my new monitor on system startup, so I don't have to think about it and hopefully it will just hum away logging what's going on and letting me know when something interesting happens.
If you're in the space you may wonder why I didn't integrate with Home Assistant. The answer is that Home Assistant is cool and open source and stuff but... not really up my ally. It feels large and bloated, it's nearly impossible to run without virtualisation, it's intended for people who like clicky-button interfaces rather than configuration-as-code, and I just don't need it. Having Home Assistant play middleman would, if anything, obscure the simplicity of what's going on here, adding layers of abstraction, conversion, protocols, DSLs that would be in my way.
FOLLOWUP
I've been running this software and indeed it is more stable than my python version. It took me about 3 days to write. By the time I had all the features and it felt like it was working, it was working. After that I had one bug in room joining that was a pure logic error on my part, and that was it. I added a few more design improvements and adjustable values, but it was a drastically different experience from the weeks of bugs still popping up occasionally in the python version.
I noticed that the development process in rust is very different. Before retiring recently my job was coding in C++. I'm not an "outline" kind of person. I'll start in one of two places. If I'm worried that something is going to be too hard or impossible, I'll start there to gain clarity on that piece of the system and "de-risk" the project. If I'm not worried and I'm fuzzy on the overarching design I'll start with the part I do know so I can build outwards from there. Both of these approaches work well in the languages I've used the most professionally: C++, python, javascript, and a number of DSLs, but rust has it's own ideas.
In rust most of your time is spent trying to get the program to compile. I'm a newbie so this is extra true for me, but I have enough experience in languages like SML and Ocaml to have a good idea that this remains true even for fairly skilled developers. In fact, arguing with the compiler constantly is the point of rust. You spend your time trying to get it to compile, possibly re-writing it a couple of times as your realize your way of thinking of the problem isn't compatible with rust's, instead of spending time debugging. The compiler is telling you "no" and forcing you to work through things you wouldn't realize until testing in other languages. That's a wonderful property. There's a side-effect though where you quickly find you're constantly asking "will the compiler let me do that". To answer that you need to be able to ask, and for to ask it the rest of your program needs to be valid. So, there's this strong incentive to keep the whole thing compilable most of the time as you develop. For this reason I quickly found myself switching to an outline style of programming. I'd write a "dummy" for a function or a struct so I could write outer code first, then go write the inner code later. This isn't a bad thing, but it's the first time I've run into a language who's design so strongly encourages one approach over another.
Linux Software I use
2023-03-24
I find that a large percentage of my time "tweaking" linux is spent searching for software that I like. I've slowly built up a toolset that's lightweight and stable, and does the things that I generally need to do - and I thought that *maybe* someone else would find this list useful too. I am sure there are better ways to do some things, but I'm pretty happy with most of how my setup works.
Rather than try and come up with a list of programs I use, Gentoo already has this list sitting around for me in the form of the world file. For non-gentoo folks, the world file is a list of every package I explicitly installed, and doesn't include dependencies. So it's pretty much everything I use. I did drop some stuff from the list that's just boring, like fonts and git.
One reason this list might be interesting to someone is that this configuration is 100% wayland. I'm not running X or XWayland. So if you're looking for a wayland solution to something, this list might be a helpful list. Another reason is that I strongly dislike heavyweight software. I will generally choose the lightest-weight option available. I do still have thunderbird on this list, I'm not saying I always use the most minimal option. Sometimes I just want to look at a darn graphical calendar, but the bias in this list is clear.
Lastly you may notice that my configuration isn't over-engineered, in fact it's very under-engineerd. I have hard-coded paths just written into scripts. This is partly because this really is what I run, not some cleaned up configuration I created for posting. It's also because I don't like over-engineering. As is I can fix this stuff really easily. My notifications aren't working? Oh yeah, that wav file doesn't exist, eh, just find a new one. It's no harder to modify notifications.py than it is to modify the config that calls it. I'm probably erring too far on the side of lazyness, but hey, it works.
I've dropped some of the less interesting entries from the world file, things like git, fonts, etc.
- app-admin/keepassxc - password DB
- app-editors/vim - preferred editor
- app-laptop/laptop-mode-tools - preferred power managemenet
- app-misc/evtest - for UI tweaking, tests for events
- app-misc/jq - for commandline fiddling with json
- app-misc/khal - commandline calendar
- app-misc/khard - commandline contacts
- app-misc/ranger - commandline file browser
- app-misc/rdfind - find duplicate files
- app-misc/screen - useful for ssh access mostly
- app-office/abiword - reading microsoft .doc files
- app-office/gnumeric - spreadsheets
- app-portage/cpuid2cpuflags - identify flags for compiling
- app-portage/eix - find packages to install
- app-portage/genlop - examine build speeds and such
- app-portage/pfl - find what packages owns a file
- app-shells/gentoo-bashcomp - bach completion is nice
- app-text/antiword - make .doc files text files
- app-text/calibre - ereader (with DeDRM ability)
- app-text/xournalpp - PDF reader/editor
- dev-python/vdirsyncer - syncs data for khal,khard, and todo
- games-strategy/wesnoth - a fun game when I'm bored
- gnome-extra/nm-applet - network control
- gui-apps/clipman - better copy/paste semantics
- gui-apps/foot - terminal, I use the client/server mode, because it saves ram
- gui-apps/nwg-launchers - a nice app-grid, mostly used on my phone
- gui-apps/swaybg - set sway background
- gui-apps/swayidle - lock sway
- gui-apps/swaylock - lock sway
- gui-apps/tiramisu - notification middle-ware, I use a python script to take the output
- gui-apps/waybar - a taskbar, tray works where swaybar tray doesn't (in gentoo add the tray use flag)
- gui-apps/wlr-randr - change display settings
- gui-apps/wofi - another menu program, used for power menu on my phone
- gui-wm/sway - WM
- mail-client/mutt - email
- mail-client/thunderbird - gui email + calendar + rss reader, sometimes guis are nice
- media-gfx/geeqie - image library viewing
- media-gfx/imagemagick - image conversion
- media-libs/libsixel - allow w3m to show images in foot
- media-sound/abcde - rip CD music (I have a USB drive for this).
- media-sound/clementine - music player
- media-sound/fmit - guitar tuner
- media-sound/lingot - another guitar tuner I'm trying
- media-sound/pavucontrol - volume control
- media-video/mpv - video player
- media-video/pipewire - sound daemon
- media-video/vlc - old video player I'm moving off of
- net-fs/sshfs - to mount my servers FS if I want
- net-im/nheko - matrix chat client with E2EE
- net-irc/irssi - IRC client
- net-mail/grepmail - for looking through old mail archives
- net-misc/networkmanager - networking (matches nm-applet)
- net-misc/nextcloud-client - file sync
- net-p2p/qbittorrent - download stuff
- net-voip/linphone-desktop - SIP client
- net-vpn/wireguard-tools - VPN to server
- net-wireless/bluez-tools - bluetoothctl to manage bluetooth
- net-wireless/iw - wireless management
- net-wireless/kismet - wireless debugging
- sci-visualization/gnuplot - making graphs
- sys-apps/ripgrep - fast grep
- sys-power/acpi - get battery state
- sys-power/cpupower - CPU frequency scaling
- sys-power/hibernate-script - hibernation
- sys-process/iotop - IO load
- sys-process/lsof - find programs with open files
- www-client/chromium-bin - spare browser (when firefox fails to work)
- www-client/firefox - primary browser (I secure this one)
- www-client/w3m - console browser, and viewer for mutt
- www-misc/kiwix-desktop - wikipedia offline
- x11-misc/gmrun - app launcher
Not every piece of software I use is in the portage package system (or maybe it is but I haven't got searching for the overlay). I should probably write ebuilds for these and be all cool and Gentooy, but I haven't.
- itd - for syncing my pinetime watch
- todoman - for managing tasks (synced by vdirsyncer)
- gauth - a little TOTP tool written in go, haven't fully moved to keepassxc
Then I run a few things on my server:
- Nextcloud - for calendar, tasks, and contacts
- Nextcloud news app - for reading news
- Dovecot+fetchmail - pop email from gmail and serve it
- dnsmasq - to redirect my domain internally
But... how do you configure sway you ask? Never fear, here's my sway and waybar configs from my laptop. It's a pretty vanilla config, not much to see here. The notification bits are probably the most interesting
- waybar config waybar styling
- sway config Note: this is from my laptop and written for keepassxc to be the secrets provider (which I don't actually recommend). I'm using gnome-keyring as a secrets provider on my phone, and using systemd there. I also have some extra input/output statements to rotate the display and set up the pinephone keyboard. On openRC I start this with `exec dbus-run-session sway`, on systemd it's just `exec sway`.
- notifications.py is a python script that processes the output of tiramisu and dumps the result to an ephemral foot terminal. This is how I do notifications. The reason I do it this way is so I can make a sound when I get a message in nheko, so I don't accidentally ignore my S.O. This script just hard codes that to an "ugg" sound from wesnoth. Any old wav file will do. Note that foot is started with a special flag so sway can decorate it differently, and let it float.
Then a couple of short scripts I'll just post inline. The volume script is interesting because it changes ALL the pulseaudio volumes. This means shortcuts using it that get attached to e.g. the volume buttons on my phone, work when using plug-in headphones, a bluetooth speaker, USB headphones, or the built-in speaker. I've tried a lot of scripts over the years and this works the best so far. It DOES occasionally cause a weird jump in the settings if something else touched a volume, but I prefer everything to just adjust together for simplicity
volume
change=0
device=alsa_output.pci-0000_00_1f.3.analog-stereo
cur_vol=$(pactl get-sink-volume ${device} | awk '/front-left:/{gsub("%",""); print $5}')
new_vol=$((cur_vol + ${change}))
echo $new_vol
pactl list sinks | awk '/Name:/{print $2}' |
while read SINK; do
pactl set-sink-volume $SINK ${new_vol}%
done
lcd_brightness
#!/bin/bash
v=$(cat /sys/class/backlight/intel_backlight/brightness)
expr $v + $1 >> /sys/class/backlight/intel_backlight/brightness
It's possible to get w3m to display images in foot, even over ssh, which is pretty cool. I can run mutt on my server (I have it both places) and view images in an email if I want! This took a lot of poking around the internet, digging through configs, and guessing to figure out - and I've not seen anyone mention it anywhere, so I'm going to add it here. To convince w3m to display images in foot select "img2sixel" for the "inline image display method". For that to work img2sixel needs to be installed, which is part of libsixel in Gentoo. I have w3m-0.5.3_p20230121 built with imlib, gpm, ssl, and unicode use flags. I also have fbcon set, but I'm 95% sure it's not needed. As long as the terminal supports sixel (a format for displaying images in terminal emulator) it'll work. If it's working w3m www.google.com will display the google logo as an image.
PinephonePro with Gentoo
2023-03-22
In this post I'm going to skip the why and jump to the what. I crosscompiled Gentoo for my Pinephone Pro with Pinephone keyboard, and got it working pretty nicely, and I wanted to document some of the process for others.
Obligatory photo of phone in operation
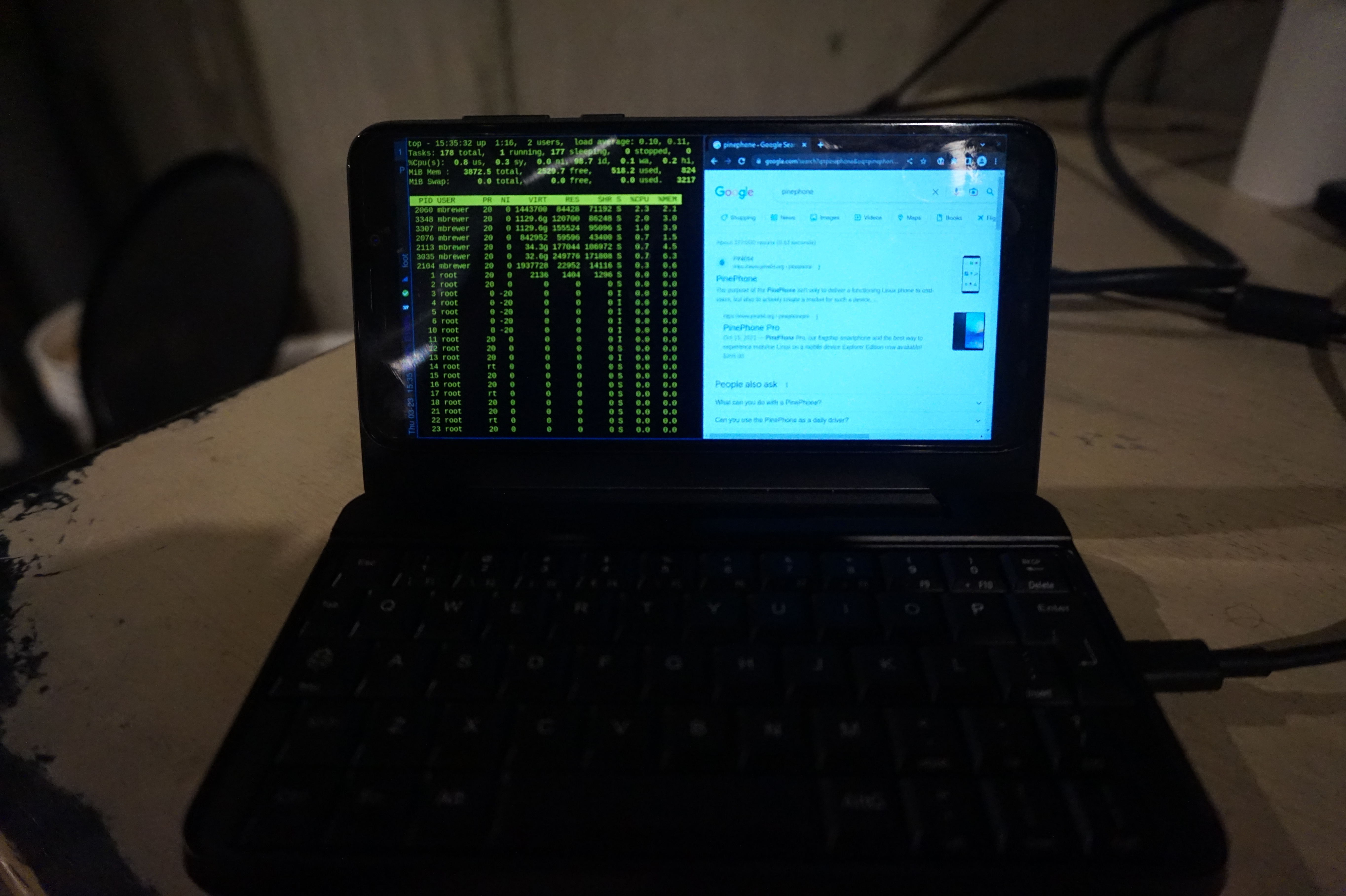
In retrospect the easiest path to get close to what I'm running would be to install mobian and then just use sway instead of phosh etc. One advantage of Gentoo though is the ability to run wayland-only which saeves on resources. An easier way to get Gentoo would be to just download an arm64 stage 3, downlad a mobian image, replace the userland with the stage3 tarball, and you'd be most of the way there. That is not the path I took though. One downside of that approach is that you aren't left with a matching cross-compiler environment for building packages requiring more than the 4GB of ram on the Pinephone Pro. Whether it's worth the extra effort, is up to you.
What is this post's purpose? I'm an experienced software engineer and long-time Linux user and admin. I've run Gentoo in particular before, and recently came back to it. That said, this was my first time building or using a cross-compiler or doing any sort of project like this. My target audience is folks similar to myself. The following is not intended as instructions for beginners. It's an approximate outline that I hope can help others with similar experience avoid a lot of dead ends, and many many hours of googling, or enable someone with a bit less knowledge to pull this off at all.
- What works: Everything you'd have on a laptop
- What doesn't: Everything you wuoldn't.
I'm kind of joking, but so far I haven't tried to get GPS, or the cell modem to work. My priority was to make it useful like a laptop. I have tried to get the camera to work. The client software is called "Megapixels". It installs fine, and I've heard works on the pinephone, but the pinephone pro needs some kernel patches that are not widely used yet. I DO have full convergence working with the kernel config I posted. I can use a USB or bluetooth keyboard, a USB-C HDMI hub, an external HDMI monitor, a USB keyboard, and an ethernet device built indo the USB-C hub, and it all works great. My bluetooth headphones work well, my USB headset works fine. I have the power-button suspend via elogind. My volume buttons work. I can change screen brightness with keyboard shortcuts. If you DO use my config and do anything I don't, you'll probably need to enable those features :).
Honestly, if you manage to truly brick your phone I'm impressed, it's not easy with the pinephone pro... but should you pull it off you're doing this at your own risk, I'm not liable, not my fault, bla bla bla. This just my blog. I don't know what I'm doing.
-
Step 1: Build a crosscompiler.
emerge crossdev and, assuming you want a gnu userland, run
`crossdev --stable --target aarch64-linux-gnu`
When your done you'll have a bunch of tooling with the prefix `aarch-linux-gnu` e.g. `aarch64-linux-gnu-emerge`. These are all your cross-compilation tools. I recommend skimming them to see what you've got. /usr/aarch64-linux-gnu will contain the new userland your building
-
Step 2: Configure make.conf
Configure make.conf for your new userland (found at /usr/aarch64-linux-gnu/etc/portage/make.conf).
Here's what I'm using for CFLAGS and CXXFLAGS. Apparently "neon" and floating point is implied already by it being armv8. From what I've read the pinephone pro has 4 cortext a72 processors and 2 cortex a53 processors, so that's why the weird flags here. You can probably do better for for rust. Lastly CFLAGS_ARM is directly from cpuid2cpuflags, but I'm giving it here so you can use it before getting something running on the pinephone.
COMMON_FLAGS="-O2 -pipe -fomit-frame-pointer -march=armv8-a+crc+crypto -mtune=cortex-a72.cortex-a53" RUSTFLAGS="-C target-cpu=cortex-a53" CPU_FLAGS_ARM="edsp neon thumb vfp vfpv3 vfpv4 vfp-d32 aes sha1 sha2 crc32 v4 v5 v6 v7 v8 thumb2"
I highly recommend you enable buildpkg in FEATURES now. This way everything you build will also get packaged and set aside, allowing you to use this machine as a gentoo binhost to re-install the packages later on without rebuilding them.
-
Step 3: emerge @system.
You'll find a lot of circular dependency problems and the like if you mess with your use flags at all. I solved these by flipping flags back off again, and then back on and rebuilding again. e.g. I want a standard pam install, which requires building without it, then turning it on and rebuilding some stuff with --newuse. Just iterate 'til you get a userland with the functionality your looking for.
-
Step 4: qemu
At this point I would highly recommend that you install qemu and set up a kernel feature called binfmt_misc on the build host. This will let your x86_64 CPU run binaries intended for your aarch64 pinephone pro, albiet slowly. This lets you test things, and "natively" compile stubborn packages that refuse to properly cross-compile, allowing an end-run around those problematic dependencies that otherise stop you in your tracks. You can just build most packages on the pinephone of course, but the ability to use a desktop-system's ram can be useful at times.
Install qemu on the host system, with aarch64 support (controlled by use-flag). Start the binfmt service (which is part of openrc), and then register qemu for aarch64 binaries.
/etc/init.d/binfmt start echo ':aarch64:M::\x7fELF\x02\x01\x01\x00\x00\x00\x00\x00\x00\x00\x00\x00\x02\x00\xb7\x00:\xff\xff\xff\xff\xff\xff\xff\xfc\xff\xff\xff\xff\xff\xff\xff\xff\xfe\xff\xff\xff:/usr/bin/qemu-aarch64:' > /proc/sys/fs/binfmt_misc/register
And then try and run any binary from your new build (in /usr/aarch64-linux-gnu). Something like `ls` is a good choice.
-
Step 5: emerge some other stuff.
It's nice to have network working, so you can install more stuff on the pinephone pro. I decided to use networkmanager, figuring i could use nmcli to get things going, and nm-applet would be nice once I had sway working (and it does work well). Your favorite editor (I use vim). Console tools, maybe dosfstools, all that stuff that's annoying if it's not there from the getgo.
In my case I didn't have the pinephone yet, so I started building all sorts of things I wanted. You can do this, but don't expect all packages to work. The bulk of packages will cross-compile fine, but some won't, and a few will appear to work fine but actually spit out an x86_64 binary (which is rather less helpful). You can fight your way through getting some stuff to work by installing things on the host system, e.g. nss, the ssl library, works fine as long as it's also installed on the nhost. It's messy - but it's also useful if you need some larger packages that won't build well directly on the pinephone.
If you get stuck and can't cross-compile something, there's a trick. We can run aarch64 binaries, including the compiler, so we can compile "natively" (as far as packages are concerned) on our host system. This can get you out of a jamb if you're trying to build something on the host system. To do this go to Step 5, then come back.
-
Step 6: chroot into your new userland
For this just go to the Gentoo Handbook and follow the instructions. They are more correct and complete than anything I can write.
etc/portage/make.conf has some hard-coded paths relative to the host OS in it. You'll need to change these before you can use "emerge". I think I've seen some ways to be able to cleanly switch between cross-compiling and local "native" compiling in qemu, but I haven't run them down yet. Anyway, I just commented thse lines out e.g.:
#CBUILD=x86_64-pc-linux-gnu #ROOT=/usr/${CHOST}/ #PKGDIR=${ROOT}var/cache/binpkgs/ #PORTAGE_TMPDIR=${ROOT}tmp/ #PKG_CONFIG_PATH="${ROOT}usr/lib/pkgconfig/"
We're note quite done building our userland image yet, but we're close. We *could* build everything ourselves, but to get things up and running a piece at a time it's easier to steel them, and then go back. So, we're going to jump to setting up our SD card, so we can steel things from mobian.
-
Step 7: Test your phone, and sdcard, with mobian
Download a mobian image, install it on an SD card, and make sure it boots. The last thing you need is thinking your image is bad, and it's actually your phone. This ensures both that your phone is good, and that the mobian image is good and boots. If you have trouble booting in later steps you'll be happy you proved that much was working now. Notice that the second partition in the mobian install is the root dir, and will resize automatically on boot.
-
Step 8: Finish up your base image
Put the mobian sd-card back in your phone. From the second partition steal the firmware and the modules from /lib, and copy them to the new image you've been building.
Now finish setup of your userland. You probably want to make a home dir, create a normal user, set passwords, etc. Again go back to the handbook and follow the section on finishing the install. Don't forget to enable services you want as well, e.g. `rc-update add NetworkManager default`. Consider ntp (though it won't start automatically if used with NetworkManager). sshd is a great idea, so you can work on the machine from a large keybard, and move files back and forth, to complete configuration.
Unfortunately I forgot to take notes on this, but I did have to tweak some permissions on things, and I've forgotten what. Sorry. I think it was relatively clear from the errors.
-
Step 9: Copy your base image to your SDcard
I started by deleting and recreating the second partition on the mobian SD card. Then creating a new ext4 filesystem on it. Then I copied my userland over to it.
When copying the userland there are several ways to do it correctly, and a lot more ways to do it wrong. I used `rsync` with every option in the man page to preserve things... then dropping whatever wasn't supported until it worked. `-x` is also useful to avoid copying all the virtual filesystems like /proc /dev and /sys. Another option is to tar -jxvf the whole FS and untar it like a stage3 tarball.
-
Step 10: set it up to boot
My pinephone pro was new, so came with tow-boot already installed. If you don't have tow-boot installed you should probably do that. The mobian image is set up to be booted by tow-boot. I found digging up information on bootloaders very confusing. Most of it is out of date. tow-boot seems to be the future, but no-one has documented much about using it yet (oddly). p-boot is largely deprecated now it seems, tow-boot is a fork of u-boot so u-boot is now also old news. I'm actually not sure if the mobian image has u-boot on it as a middle step, or if tow-boot directly reads the first partition - but I do know this proccedure works.
Mount the first partition of your SD card and edit extlinux/extlinux.conf. Here's what my current configuration looks like (this is booting my own kernel now). Note, the config below is for actually booting of the root filsystem, we're not quite there yet, but I wanted to post something that I KNOW is correct and functional:
default l1 menu title U-Boot menu prompt 0 timeout 10 label l0 menu label Mobian GNU/Linux 6.1-rockchip linux /vmlinuz-6.1-rockchip initrd /initrd.img-6.1-rockchip fdtdir /dtb-6.1-rockchip/ append root=UUID="503a2bc5-c313-489f-9ef2-058f2b9dc86c" consoleblank=0 loglevel=7 ro plymouth.ignore-serial-consoles vt.global_cursor_default=0 fbcon=rotate:1 label l1 menu label Gentoo GNU/Linux 6.1.12 linux /vmlinuz-6.1.12-local fdtdir /dtb-6.1-rockchip/ append root=/dev/mmcblk2p2 consoleblank=0 loglevel=7 ro plymouth.ignore-serial-consoles vt.global_cursor_default=0 fbcon=rotate:1 usbcore.autosuspend=-1
I left out the comment at the top that says not to edit this file I edited. I dropped a number of options that are just to make it pretty during boot. fbcon=rotate:1 makes it boot with the screen orientation correct for the ppkb, making it a lot easier to work with. When I tried to use a UUID on my own kernel it didn't work right, and I don't know why. /dev/mmcblk1p2 should be the correct device for the SD card, if you don't want to deal with uuids. If you do "blkid" should give you the uuids. Sadly, this does not show me a boot menu. I know some folks have a menu working, but for me it's not a big deal as I'll explain later.
-
Step 11: Boot your system
Turn on the phone (or reboot it), and the light will come on red. Immediately hold the volume button down until the light flashes blue. If it flashes white you'll have to shut it off and try again. Hopefully once you get the sequence right, it'll boot gentoo!
You can also hold the volume up button and the light will flash blue and the phone will act as a USB mass storage device. This isn't super useful now, but when you go to replace the OS on the built-in flash it's extremely useful if your phone won't boot. You can mount it on your host machine, modify things as required, unmount, and try again. With qemu set up you can even chroot into the environment if you need to. This is why I haven't found alck of a boot menu to be a big deal.
On my system the time was completely screwed up, and didn't go away until I built my own kernel. Using hwclock to manually sync the system clock after setting the clock didn't seem to help, and I don't care t ogo back and understand since it all works now. In any case you may have to set the time using `/etc/init.d/ntp-client restart` to get some things to work like validating ssl certs
You're now about to find out that the pp keyboard won't let you type some of the charactors like '-'. Ooops, lets go fix that. The xkb mapping is actually probably already installed.
-
Step 12: Make the PP keyboard work
Here's how to fix it under console, via loadkeys https://xnux.eu/pinephone-keyboard/faq.html
For sway you can just add this, which will just tell it to use the pinephone keyboard mappinginput "0:0:PinePhone_Keyboard" { xkb_layout us xkb_model ppkb xkb_options lv3:ralt_switch }
About here I actually paused to get things set up how I actually like them. I got sway installed, a browser, and all that nice stuff. You CAN just stop here if you want in fact and skip Steps 13 and 14. It's totally up to you. Regardless you might want to skip down and read the more open tips section of this post, and come back when you're ready to commit to this OS on your phone.
- Step 13: Copy to your root system if you want to Once you are happy with your system you probably want to put it on pinephone itself. There are lots of ways to do this, personally I just repeated the trick with the mobian install, then copied the data from my system partition over using the same rsync trick. Again you have to edit the boot options for extlinux.conf. `/dev/mmcblk2p2` is what you'll want for the root device, I couldn't get UUIDs to work for some reason. If you render the phone unbootable at this stage, that's fine. Remember that the volume-up button will make your phone into a mass storage device, mount it on your other machine over USB, and just fix your mistake.
-
Step 14: Build your own kernel
The "bingch" overlay has a lot of pinephone stuff, including firmware and kernel source. If you want to run phosh it's in there too.
My kernel config for the linux-orange-pi-6.1-20230214-2103 from the bingch overlay has most things built in and doesn't require an initrd/ramfs. I started from the mobian config, but if your looking for a more minimal starting point my config is here . I make no claims as to the appropriateness of this kernel, kernel options, whatever, all I can say is I'm using it and it seems to work well.
obligatory photo of pinephone pro doing convergence stuff
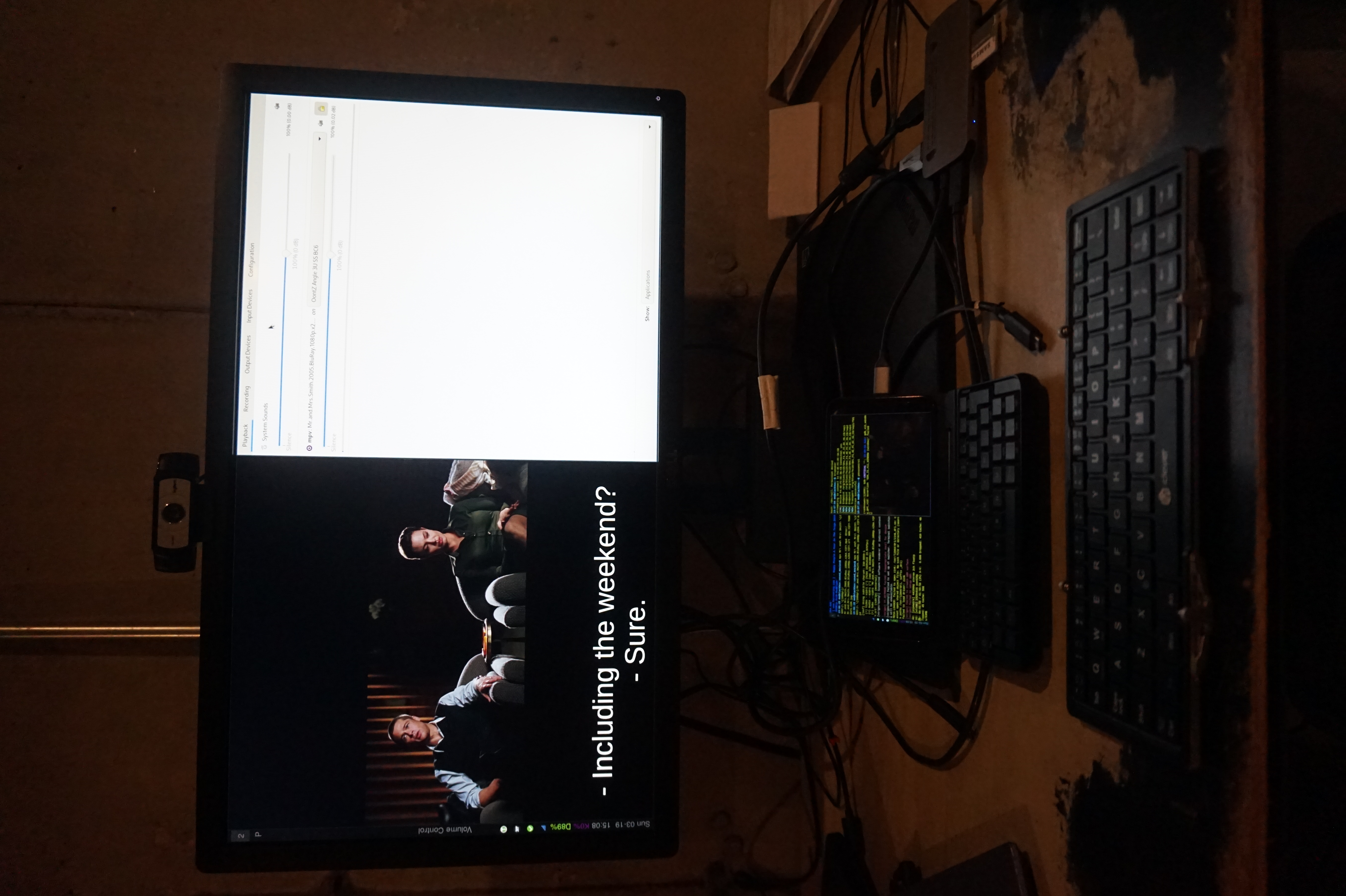
Pinephone Tips
At this point hopefully you have gentoo booting on your pinephone. But that's a ways from having everything you want working. Since we've basically set up the pinephone like a laptop you can test things out on a laptop and then apply the changes to your pinephone if that's helpful. But here's some ideas
As I mentioned I'm using wayland only swayWM. Some software I like
- nwgrid is a nice standalone grid for app launching
- foot is a lightweight terminal (especially in server/client mode)
- puremaps and OSMScoutServer for offline maps are available via flatpaK
-
waybar with the tray enabled displays things like keepass and nm-applet nicely. It also has the ability to display what's going on with multiple batteries. For the config I added this
"battery": { "bat": "rk818-battery", "format": "D{capacity}%", "format-charging": "D{capacity}%", "rotate": 90 }, "battery#keyboard": { "bat": "ip5xxx-battery", "format": "K{capacity}%", "format-charging": "K{capacity}%", "rotate": 90, },
and for the style.css I added this
#battery { color: #7b0075; } #battery.charging { color: #7bc200; }This allows me to tell the state of the keyboard battery, if I disable it the displayed charge will drop to zero. This is useful because only in this state will the device turn off, otherwise it detects the keyboard as a powersource and turns on so it can manage charging. The pinephone pro still lacks firmware capable of doing this charging logic, so it boots all the way up into your OS. So understanding what's going on with the battery is key to making good use of the ppkb.
- Sadly firefox-bin is x86_64 only currently, but chromium-bin installs fine. Everything works but widevine.
- rust-bin will also save you some time compiling
- This isn't pinephone specific. swaylock should be setgid shadow apparently, but I don't have a shadow group (I probably should), so setuid root at least works :(.
- I log in from console and have .bash_profile check for SSH_CLIENT, if it's not set it starts sway. This makes login quick and few keypresses. I also have gnome-keyring configured as part of pam so it'll fire up as sway starts, this way e.g. my encrypted chat just opens and works without typing more passwords. I don't care on desktop, but when I turn on a phone/PDA I probably want to use it NOW to write something down, snap a photo, whatever.
-
swaymsg output DSI-1 scale $1
Is a useful little script for a pinephone. In wayland you can rescale the entire interface. I keep it set to 1, but 1.5 is nice occasionally.
I'm hoping to write up another post about my desktop configuration and using sway soon, for less pinephone specific information. But there you go.
This is not the easiest process, but compared to your average "build your own OS for arbitrary device" project I suspect this is downright trivial. If you're good with linux and understand how it all works this actually isn't all that hard. I had to do a lot of reading to e.g. work out what the cflags should be, which bootloader to use, the best way to get a bootable system, etc. Hopefully this set of psuedo-instructions will save you those headaches and make the project only a little more involved than a typical gentoo install.
Lastly, other people blazed this trail already. First the all the folks who patched the Linux kernel, wrote firmware for the pinephone keyboard, etc. And then folks who build Gentoo for it before me. I just followed in there footsteps. https://gitlab.com/bingch/gentoo-overlay/-/blob/master/README.md is the best resource I found https://xnux.eu/howtos/build-pinephone-kernel.html Is where I found megi's sources (though I ended up using the ones from bingch). Megi did a lot of the work of writing patches and collecting disprate patch sets together to get the pinephone to really work well. I know I used a couple of other sources for gentoo-specific pinephone knowledge, but have forgotten what they worry, so appolagies for not citing you, whoever you are.
String Sorts
2019-01-23
You'll recall in my recent post about Fast Sort we learned that Radix Sort is significantly faster at sorting 32 bit integers than normal comparison based sorts, particularly as the array gets large. A result every computer scientist knows theoretically, yet with median-find we saw how theoretical bounds don't always translate in practice.
Well, a friend asked me, what about sorting variable length strings? Does radix sort still beat comparison based sorts? It's a fair question so, I decided to take a shot at it. And here are my results:
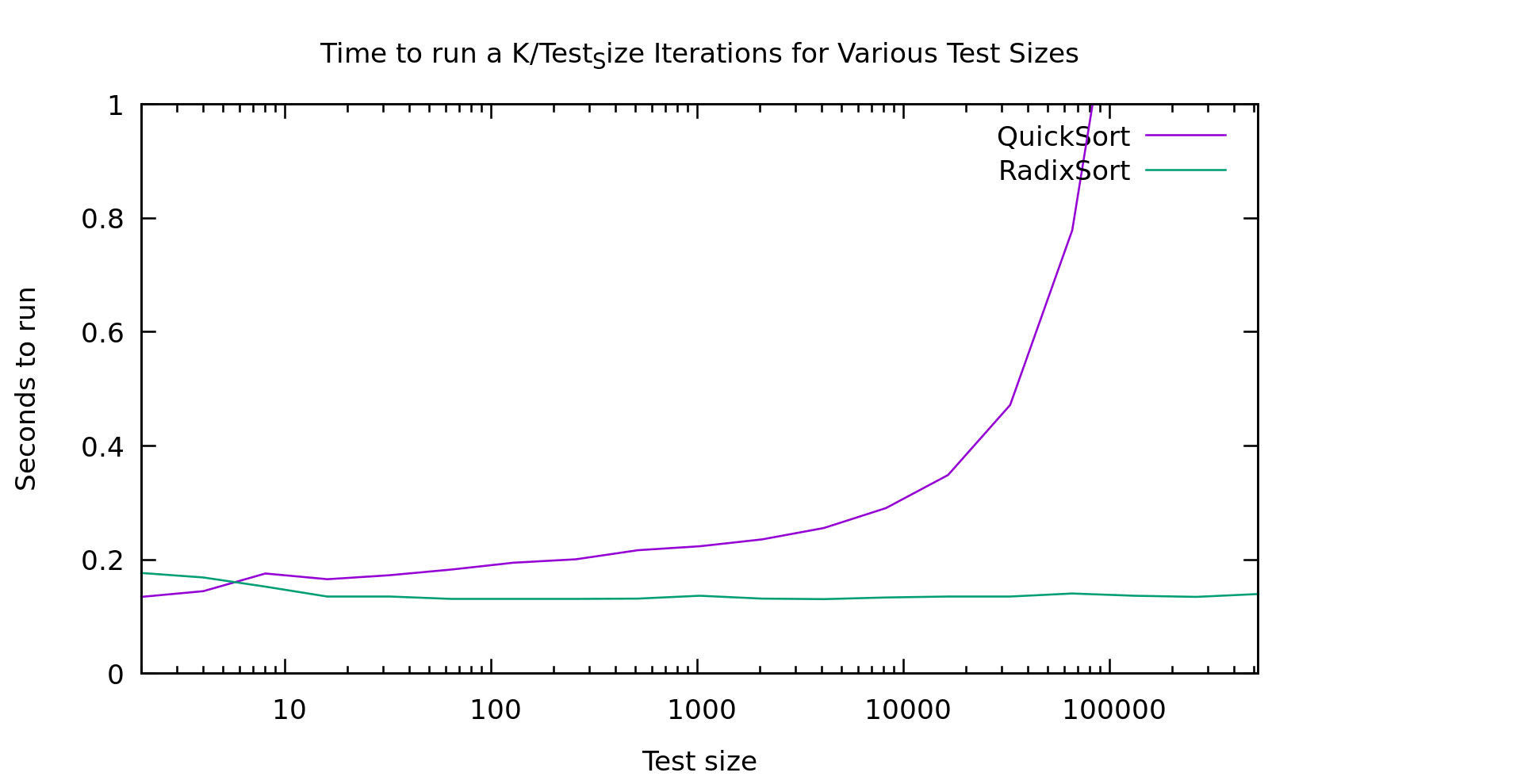
In my mind that graph is pretty clear, above 10 elements radixsort is clearly winning. Note that this is currently a 128 bit radix-sort that only handles ASCII... though I'm actually only feeding it uppercase strings currently. So, lets talk about how this algorithm works, because it's not an entirely trivial conversion of radixsort
String Radix Sort Algorithm
This is a little bit interesting. You may recall that there are two types of radix-sort. Least Significant Digit first, and Most Signicant Digit first. These are referred to as LSD and MSD. My binary radix sort from earlier benchmarks was an example of an MSD sort, and the one I just referred to as "radix sort" is an LSD sort. LSD sorts are preferred generally because they are stable, simplier to implement, require less temp space AND are generally more performant.
There's just one problem. With variable length strings, LSD sorts don't work very well. We'd have to spend a lot of time scanning over the array just looking for the longest array so we can compute what counts as the smallest significant bit. Remember that in lexicographic ordering it slike all the strings are left justified. The left-most charactor in each string is equivelent in precidence, not the rightmost.
MSD sorts, must be recursive in nature. That is, they need to work on only the sublist we sorted in to a certain bucket so far. I'm going to call this sublist a "slice". To keep our temporary space vaguely in order I'm using a total of 5 lists.
- The input list of strings (call this string list A)
- Temporary list of strings (call this string list B) (length of A)
- Temporary list of indices into string list A (call this slice list A) (length of A)
- Temporary list of indices into string list B (call this slice list B) (length of A)
- An list of 129 buckets
Here's the algorithm. Start by looking at the first bytes of the strings. Look in slice list A, and get the next slice. Bucket everything in this slice. Each of these buckets (if non-empty) becomes a new slice, so write strings back out to string list B, and write the index of end each slice in to string list B. Swap lists A and B, move to the next byte, and do it again. We terminate when for each slice it's either of length 1, or we run out of bytes. To see the full implementation take a look at string_sort.h in my github repo .
Conveniently, they way my algorithm works it is in fact stable. We walk the items in order, bin them in order, then put them in the new list still in order. If they are equal there is no point where they'd get swapped.
It's a LOT of temporary space, which is kind of ugly, but it's pretty performant as you saw above. Another optomization I haven't tried is short-circuiting slices of length 1. We should be able to trivially copy these over and skip all the hashing work. Testing would be required to see if the extra conditional was worth it... but It seems likely
Data tested on
To test this I'm generating random strings. It's a simple algorithm where, with a probability of 9/10 I add another random uppercase letter, but always stopping at 50 charactors. I'm mentioning this because obviously the distribution of the data could impact the overall performance of a given algorithm. Note that this means functionally we're only actually using 26 of our 128 buckets. On the other hand, real strings are usually NOT evenly distributed, since languages carry heavy biases towards certain letters. This means my test is not exactly represenative, but I haven't given it a clear advantage either.
Conclusion
I can't say that this is a clear win for Radix Sort for sorting mixed-length strings. The temporary space issue can be non-trivial, and certainly time isn't always worth trading for space. We're using O(3N) additional space for this sort. That said, there are some obvious ways to reduce the space overhead if you need to, e.g. radix-sort smaller chunks of the array, then merge them. Use 32 bit instead of 64 bit pointers, or come up with a cuter radix-sort.
Note that my radix-sort was a mornings work to figure out the algorithm, write and validate an implementation, find a couple optomizations, and benchmark it. I wrote this post later. Oddly "inline" made a huge difference to gcc's runtime (it's needed due to loop unrolling for handling the A/B list cases). In any case, I've little down someone can beat my implementation, and maybe find something using a bit less space. I just wanted to prove it was viable, and more than competitive with comparison based sorts.
Median Find
2018-12-19
Similar to Radix Sort, I thought it might be interesting to see how worst-case linear time medianfind actually performed. Since the algorithm is identical to expected-case linear-time medianfind (usually called quickselect), except for the pivot selection, I elected to add a boolean to the template to switch between them (since it's in the template it'll get compiled out anyway). Before we go in to the results, here's a quick refresher on these algorithms:
Problem Statement
Imagine you have a sorted array. If you want the K'th largest element, you can simply look at the element in location K in the array. Comparison-based sorting an array takes O(Nlog(N)) time (strictly speaking the theoretical limit is log-star, but it doesn't really matter). What if we want to do this without sorting the array first?
Quick Select
Quick select chooses a pivot and runs through the array throwing elements in to 2 buckets... smaller and larger thanthe pivot. Then it looks the number of elements in the buckets to tell which one contains the k'th element, and recurses. We can prove we usually choose a good pivot and this is expected O(N) time. But it's worst-case is much worse.
Worst-case-linear Quick Select
What if we always chose the right pivot? Or at least... a good *enough* pivot. This is how we build our worst-case-linear quick select algorithm. It's a really cool trick, but it's been covered in many places. So if you want to know how it works you can check wikipedia, or this nice explanation .
Real World performance
All of that is great in theory, but what is the *actual* performance of these things... well, in a benchmark, but at least on a real computer.
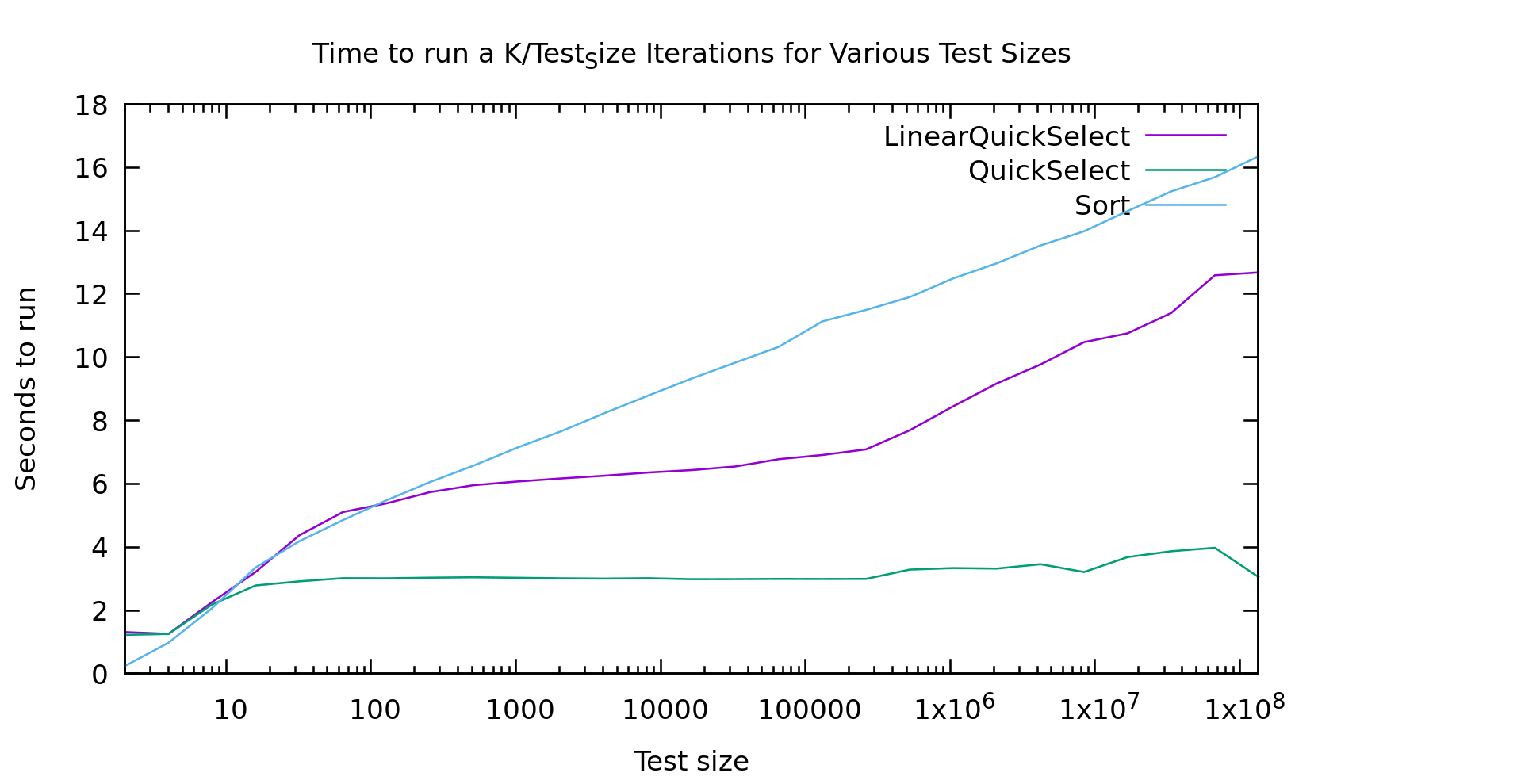
As usual I'm working on an array of test_size k/test_size times, so we work over the same number of array-cells at every point on the graph: small arrays many-times on the left, and large arrays fewer-times on the right.
For a while I was pretty upset about these results. The runtimes for "lineartime" quickselect look more like quicksort (the algorithm displayed as the "sort" line) then they do like basic quickselect. In short... that doesn't look linear at all. What the heck?
I must have a bug in my code right? This algorithm was proved linear by people much smarter than me. So, my first step was to double-check my code and make sure it was working (it wasn't, but the graph above is from after I fixed it). I double, triple, and quadrouple checked it. I wrote an extra unittest for the helper function that finds the pivot, to make sure it was returning a good pivot. Still, as you see above, the graph looked wrong.
I finally mentioned this to some friends and showed them the graph. Someone suggested I count the "operations" to see if they looked linear. I implemented my medianfind algorithm using a seperate index array. That way I could output the index of the k'th element in the *original* array. From there everything is done "in place" in that one index array. As a result, swapping two elements is my most common operation. That seemed like a pretty accurate represention of "operations". So, here's what that graph looks like.
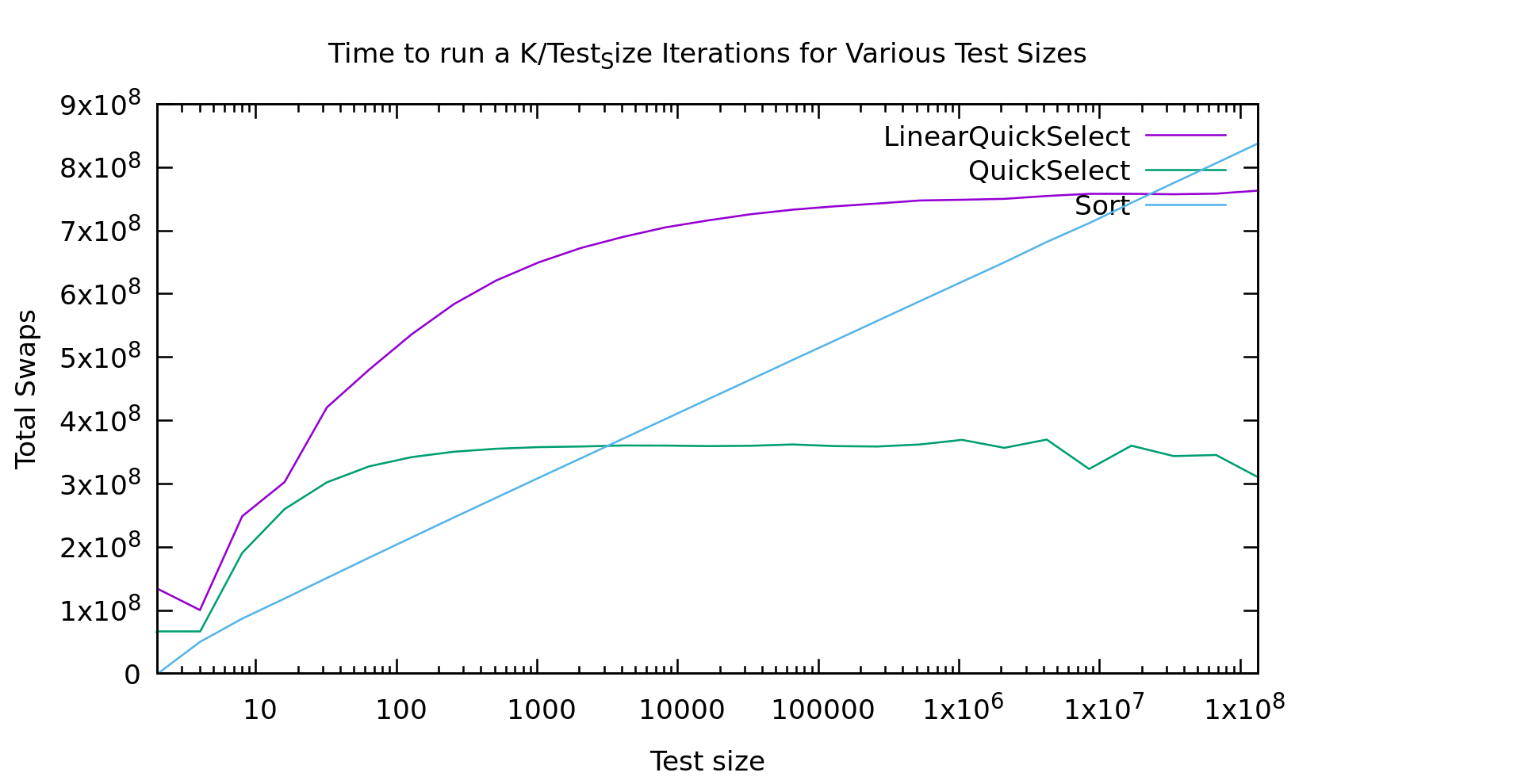
Now THAT look's a bit more linear! It's not exactly a flat line, but it looks asymptotic to a flat line, and thus classified as O(N). Cool... So, why doesn't the first graph look like this?
Real machines are weird. That index array I'm storing is HUGE. In fact, it's twice the size of the original array, because the original is uint32_t's and my index array is size_t's for correctness on really large datasets. The first bump is similar in both graphs, but then a little farther to the right in the time graph we see it go crazy... that is probably the algorithm starting to thrash the cache. Presumably if I made it big enough we'd see it go linear again. That said, if we go that large we're soon running on a NUMA machine, with even more layers of slowness, or hitting swap.
So, should you *ever* use guaranteed linear-time medianfind? Probably not. If there is a case it's vanishingly rare. It happens pivot-selection distributes well, so there's probably a use there? But, if you just used the "fastsort" we talked about in my last post you'd get even better performance, and it's still linear, AND it distributes well too! It's not comparison-based of course, but there are very few things that can't be radixed usefully with enough of them, and if you're stubborn enough about it.
Big O notation didn't end up proving all that useful to us in this case did it? Big O is usually a good indicator of what algorithm will be faster when run on large amounts of data. The problem is, what is large? Computers are finite, and sometimes "large" is so large that computers aren't that big, or their behavior changes at those sizes.